Over 3000 discrete samples of soybeans were obtained from production locations around the United States during the years 2012-2016, and from the Agricultural Research Service soybean germplasm collection. The samples were ground and scanned on two near infrared spectrometers (NIRS) and analyzed by wet chemical methods in triplicate for total carbohydrate composition (soluble sugars, total insoluble sugars, and total uronic acids) and the results are summarized in Table 1. A subset selection of these samples was used to prepare NIRS calibrations. Selected preprocessing algorithms were applied to spectral data to minimize/ eradicate noise or disturbance in the spectra. Multiple Linear Regression (MLR) analysis of preprocessed spectral data and wet chemistry data was used to develop models to predict individual chemical species. The selection of a suitable calibration model was based on a high regression coefficient (R2), and lower standard error of calibration (SEC) values. Optimized MLR regression models were then used to predict validation sets.
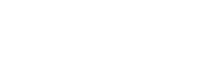